Understanding US Wind and Solar Energy Siting
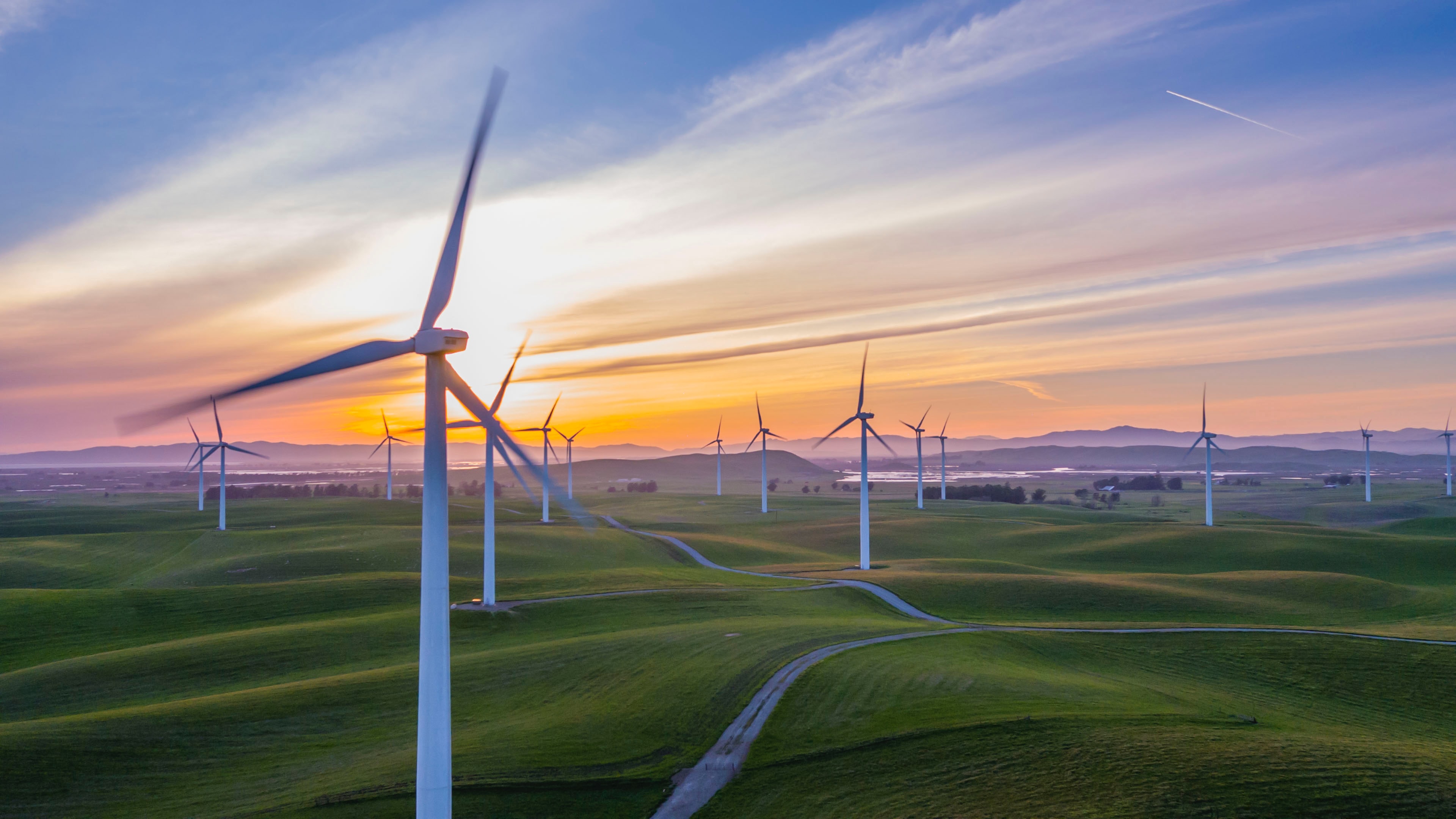
Summary: Advancing Renewable Energy Site Selection for Climate Change Mitigation
The Challenge of Decarbonization
Addressing climate change through the decarbonization of energy sources stands as one of today's foremost global challenges. The shift towards clean, renewable energy, while widely recognized as necessary, encounters complex hurdles. Despite the suitability of many regions for renewable energy, variability in their utilization and potential remains a significant issue, as highlighted in research by Pierce et al. (2021).
Understanding Site Selection
Limited understanding persists regarding the key factors that influence the successful siting of wind and solar energy projects. This knowledge gap can lead to inefficiencies or unintended outcomes in clean energy policies, as emphasized by Wu et al. (2020). Dr. Grace Wu's initial study in the western United States set a precedent for predicting energy site locations, and the objective of this expanded project is to identify the most critical factors influencing the siting of utility-scale onshore wind and solar photovoltaics across the contiguous United States.
Problem Statement: Enhancing Renewable Energy Development
Mitigating Climate Change
Rapid decarbonization of energy sources is a vital response to the escalating threat of climate change. Wind and solar photovoltaics (PV) are essential in achieving decarbonization targets, as noted by Williams et al. (2021). However, the development of these energy sources often intersects with other societal goals, such as preserving biodiversity and maintaining agricultural productivity.
Optimizing Site Selection
The process of locating suitable areas for utility-scale wind and solar PV projects requires substantial resource investment. A better understanding of the determinants for successful energy project siting can lead to more efficient electricity system planning, enhanced resource utilization, and accelerated energy source decarbonization. Additionally, the expansion of renewable energy necessitates a corresponding growth in electrical infrastructure, which typically has longer development timelines compared to renewable energy plants. Improved insights into renewable energy siting can streamline the planning of such infrastructure, contributing to a proactive approach in energy transition.
Specific Objectives: Building on Previous Research
The project aims to build upon Dr. Grace Wu's foundational study, focusing on three primary objectives:
- Data Collection and Preparation: Gathering and organizing relevant data for each factor to be included in the analytical models.
- Analyzing Relationships: Examining the interplay between renewable energy siting and various influencing factors.
- Projection Mapping: Creating maps that predict suitable sites for utility-scale wind and solar power plants, employing statistical methods and machine learning algorithms.
Testing: Ensuring Accuracy and Reproducibility
Code Testing:
- Reproducibility Checks: Ensuring scripts are operational across different operating systems, including package installation verification.
- Unit Testing: Conducting tests on data curation and combination processes for format accuracy and functionality.
- Code Review: Collaborative examination of code for accessibility and documentation integrity.
Data Testing:
- Validation and Alignment: Confirming data alignment with provided metadata and its suitability for guiding analysis.
- Type and Structure Verification: Ensuring correct data types and structures are used for calculations, and adjusting datasets to handle data gaps where necessary.
Algorithm and Analysis Testing:
- Assumption Verification: Confirming the fulfillment of regression assumptions and testing for multicollinearity.
- Model Feasibility Checks: Performing sanity checks on machine learning outputs for realistic siting predictions.
- Performance Metrics: Utilizing Receiver Operator Characteristic Curve and Area Under the Curve metrics, and implementing k-fold cross-validation for model accuracy assessment.
Acknowledgements
UCSB: Grace Wu, Assistant Professor, Environmental Studies Program; Ranjit Deshmukh, Assistant Professor, Environmental Studies and Bren School
Bren School: Niklas Griessbaum, PhD Student; Allison Horst, Assistant Teaching Professor
Lawrence Berkeley National Laboratory
The Nature Conservancy